
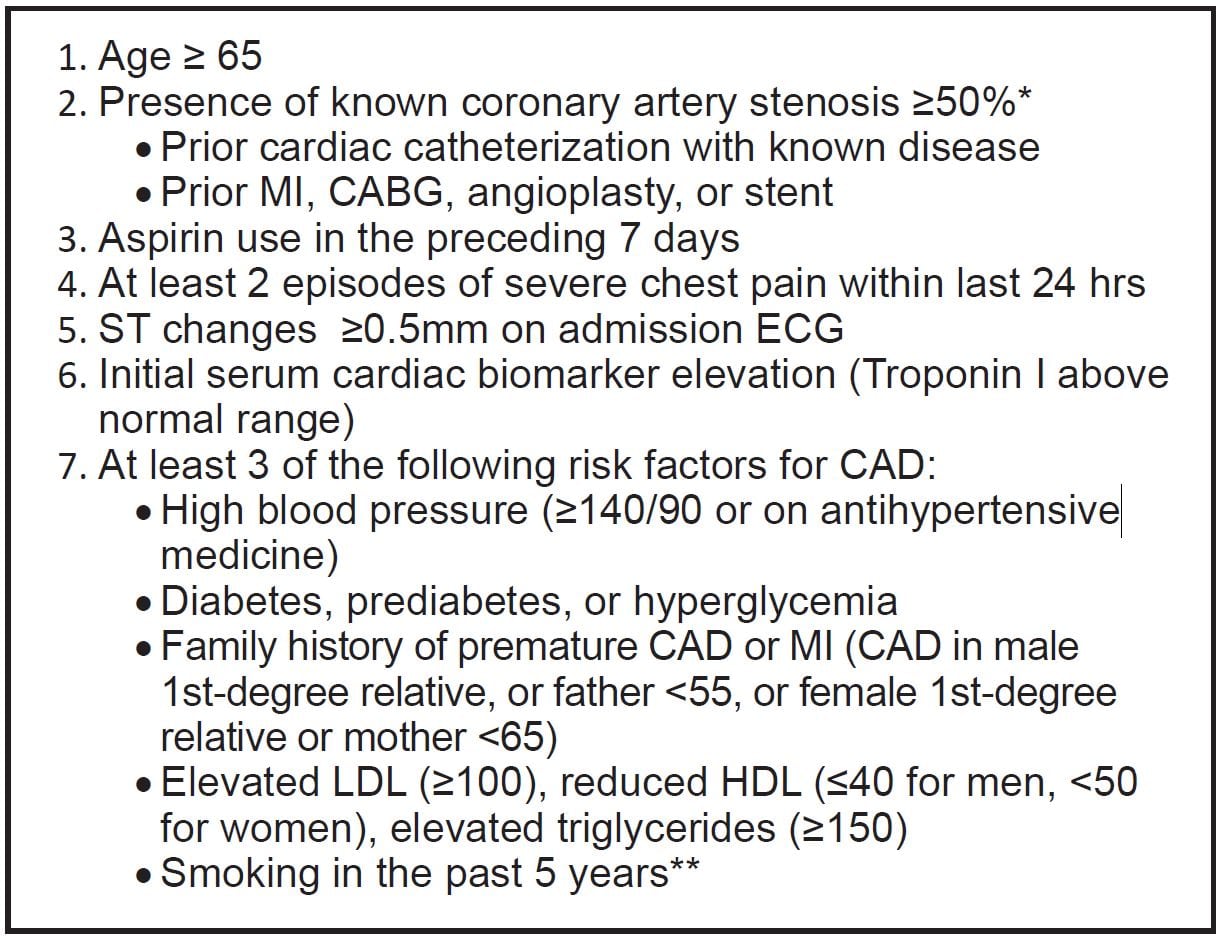
However, CV risk must be assessed when it comes to clinical decisions and interventions, not only after CV events but also to prevent them from occurring in high-risk individuals. First, healthy attitudes and behavior, which can be encouraged by low-cost measures both on a population-wide scale and aimed at high-risk patients, can render CV risk stratification unnecessary for many. With regard to the usefulness and limitations of CV risk scores and their application in clinical practice for primary and secondary prevention, it is worth examining what we know and what remains to be clarified. Using techniques from artificial intelligence, the authors performed optimization with genetic algorithms (the most popular of the larger class of evolutionary algorithms), which are among the CV risk assessment tools currently recommended by US and European medical societies (albeit without significant effect to date). were validated by k-fold cross-validation, a method for assessing the ability of a model to make generalizations on the basis of a dataset. The approaches analyzed by Paredes et al. the number of patients with the endpoint divided by the number of predictive factors under study, 3 and by this method, the frequency of the combined endpoint was low (n=33). Another important question is the frequency of the endpoint used to determine the sample size, rather than the total number of patients a simple and practical method requires at least 10 events per variable, i.e. 2 The new approaches analyzed in the study are able to cope with missing risk factors, which is a way to avoid excluding cases, although the authors recognize that care should be taken when extrapolating the results.
CAD RISK FACTORS TIMI FULL
This could be a source of selection bias, but the data on validation of the risk scores reveals that the study sample included the full spectrum of non-ST-elevation acute coronary syndromes (NSTE-ACS).
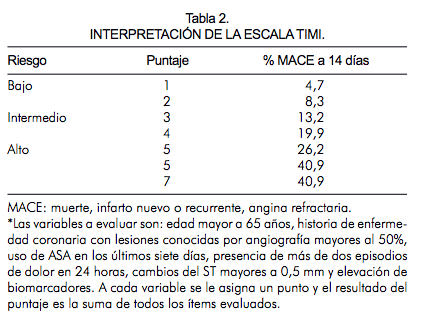
The subjects in the study were patients in Hospital de Santa Cruz, a reference center due to the quality of its interventional care, especially coronary angiography and myocardial revascularization. 1 It clearly demonstrates the need for collaboration between statisticians and clinical researchers in the development and validation of risk prediction models. in this issue of the Journal on specific aspects of statistics and information technology is an important contribution to improving risk scores for secondary prevention. The aim is to identify the main risk factors and markers that are potential therapeutic targets and to promote the implementation of cost-effective diagnostic and prognostic strategies in primary and secondary prevention of CV disease. Prediction of cardiovascular (CV) risk is an aspect of CV prevention that has seen significant developments in recent years.
